I’m a postdoctoral researcher at the Massachusetts Institute of Technology (MIT) in Boston (USA). I previously co-founded the company Yellowleaf, a digital agency, and co-led the company for nearly eight years, after which it was successfully acquired by an industry partner. I’m passionate about using technology to advance science, industry and society, and in particular how artificial intelligence and machine learning can improve our understanding of the universe and the world around us. I especially like bridging fields and integrating techniques from different domains, as I believe this is the key in developing innovative solutions to big problems.
I obtained my PhD in Artificial Intelligence for Astronomy & Astrophysics from KU Leuven (Belgium) in 2023, of which I spent one year at MIT. My academic research focuses on the development of a machine learning framework to process the millions of stars that are being observed by NASA’s Transiting Exoplanet Survey Satellite (TESS) space mission. More specifically, we first try to classify the observed stars according to their physical characteristics and then aim to determine their astrophysical parameters in detail, such that we can ultimately unravel their complete physical structure and understand the evolution of stars.
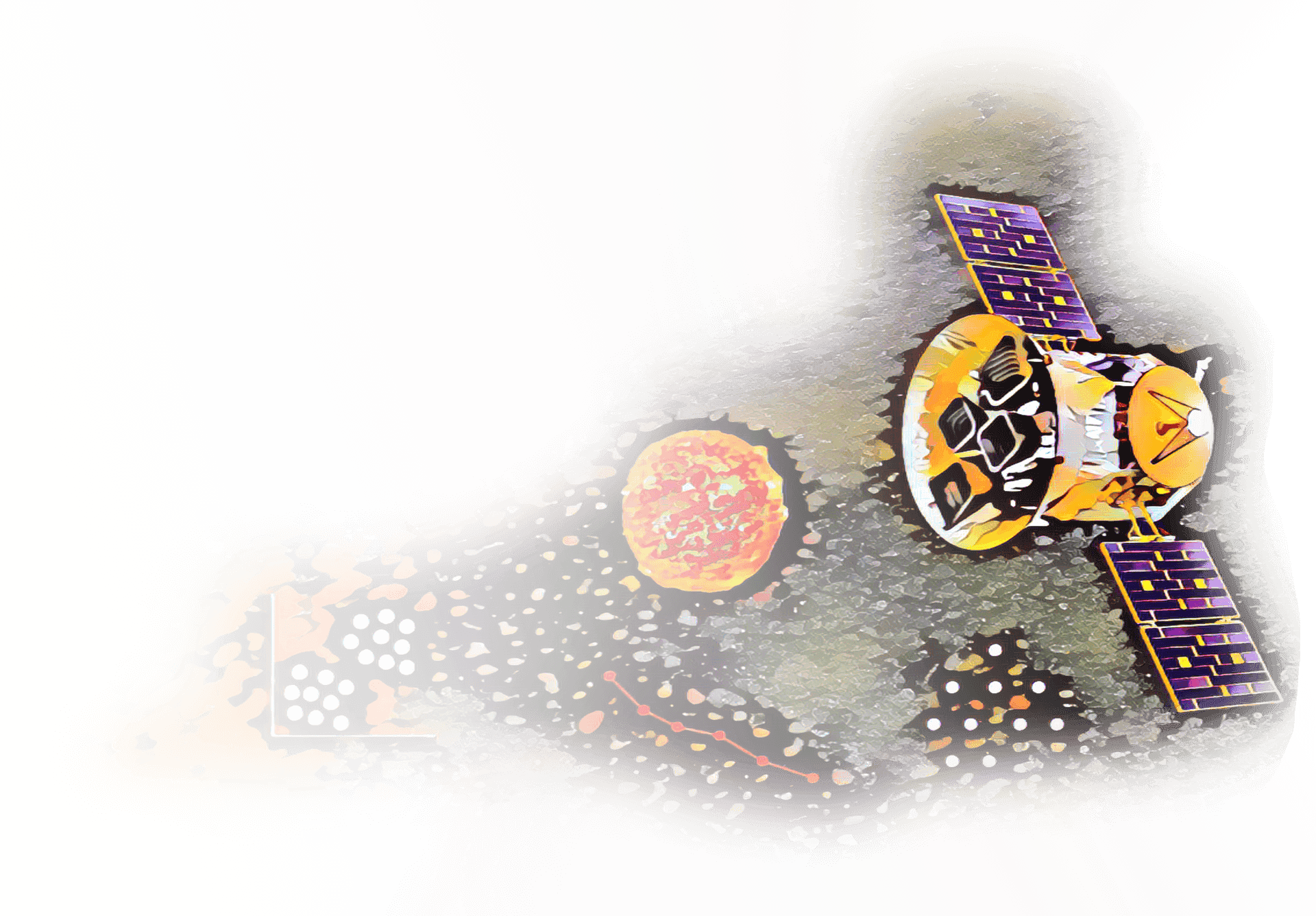
About me

Professional experience
2023 – currently, MIT (USA)
Postdoctoral researcher
2016 – 2023, Yellowleaf (Belgium)
Co-founder and co-managing partner
Academic experience
Ph.D., 2023, KU Leuven (Belgium)
Artificial Intelligence for Astrophysics
M.Sc., 2019, KU Leuven (Belgium)
Artificial Intelligence
M.Sc., 2018, KU Leuven (Belgium)
Commercial Science
B.Sc., 2017, KU Leuven (Belgium)
Commercial Science
Selected publications
Audenaert, J.; Tkachenko, A., “Multiscale entropy analysis of astronomical time series. Discovering subclusters of hybrid pulsators”, 2022, Astronomy & Astrophysics, Volume 666, id.A76, 14 pp., article link
Audenaert, J.; Kuszlewicz, J. S. ; Handberg, R.; Tkachenko, A., et al., “TESS Data for Asteroseismology (T’DA) Stellar Variability Classification Pipeline: Setup and Application to the Kepler Q9 Data”, 2021, The Astronomical Journal, Volume 162, Issue 5, id.209, 25 pp., article link
Selected talks
“Unlocking the scientific potential of large-scale astronomical surveys with machine learning”, Invited Seminar, 18 April 2024, European Space Agency
“Updates on the status of the ESA PLATO mission: asteroseismology & exoplanet science”, Contributed talk, TASC7/KASC14 Kepler/TESS Asteroseismic Science, 17 – 21 July 2023, University of Hawaiʻi, Honululu, USA
“Machine learning as a key component in the science processing pipelines of space- and ground-based surveys”, Contributed talk, Debating the Potential of Machine Learning in Astronomical Surveys, 27 Nov – 1 Dec 2023, Center for Computational Astrophysics, Flatiron Institute, New York, USA
Research interests

Machine learning
Space & astrophysics
Interdisciplinary science
Innovation
Transiting Exoplanet Survey Satellite (TESS)
TESS, the Transiting Exoplanet Survey Satellite, is an MIT-led NASA mission to discover new exoplanets, i.e., worlds beyond our solar system. The brightness measurements that are being delivered by TESS cannot only be used to find exoplanets, but can essentially be used to study almost any object in the sky that is changing in brightness over time, from nearby asteroids to pulsating stars to distant galaxies containing supernovae. Studying the host stars of exoplanets is especially important in this sense, as understanding the host environments is the key to be able to make detailed models of exoplanets.
My research in particular focuses on the study of pulsating stars or “starquakes”. Stellar pulsations are of particular importance as they can be used to probe the interior properties of the stars through which they are propagating. Given the large number observations TESS is delivering back to Earth each month, machine learning techniques are the ideal tool to process this vast number of stars efficiently and study their physical properties on a large scale.
Contact
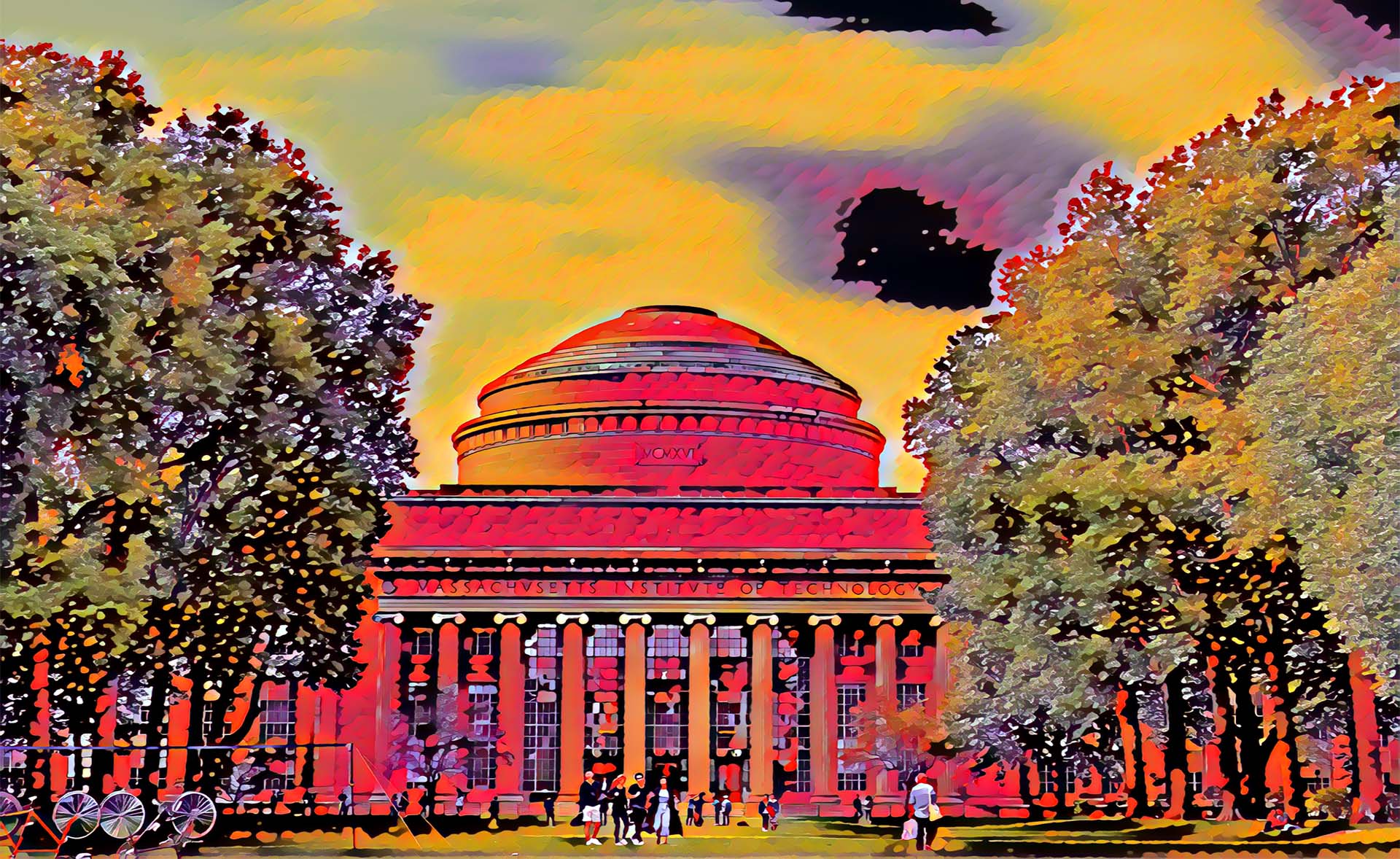
Jeroen Audenaert
MIT Kavli Institute for Astrophysics and Space Research
Massachusetts Institute of Technology
77 Massachusetts Avenue
MIT Building 37-438
Cambridge, MA 02139
jeroena (at) mit.edu